Artificial intelligence seems ubiquitous in our increasingly technology-dependent society. OpenAI’s ChatGPT, facial recognition technology, and even algorithms used for targeted ads on social media and shopping platforms are just a few examples of how machine learning has revolutionized our daily lives. Unsurprisingly, artificial intelligence has also impacted scientific investigation, including cell counting, a monotonous yet necessary task for many cell-dependent assays in the biological sciences.
According to a paper published in 2010 by Kathleen Ongena and colleagues at Millipore Inc., over 70 percent of surveyed researchers manually counted cells for assays using a hemocytometer, an instrument resembling a glass microscope slide equipped with a chamber and small grid used to aid in the counting process. Not only is the use of a hemocytometer for cell counting time-consuming and repetitive, but it also relies completely on the user, potentially introducing bias and error. In some cases, these inaccuracies in cell counting can be serious and provide misleading results that impact patient outcomes in healthcare contexts. Machine learning and automatic techniques aim to rectify these issues. What are these methods, and how do they work?

Above: Image of cells and a hemocytometer under a microscope. Image courtesy of Allevi Protocols.
Machine learning models such as U-Net, Mask R-CNN, and Feature Pyramid Network are some of the most popular methods of segmenting images, the process used to split an image into parts and identify cells. Most of these neural networks rely on a similar organization. U-Net, named for its U-shaped structure, was created by the Computer Science Department at the University of Freiburg in 2015 and uses a convolutional neural network (CNN) that is specifically structured for biomedical imaging. This intentional bio-friendly design allows users to receive highly accurate outputs with only a few training input images—unlike other more generalized segmentation models.
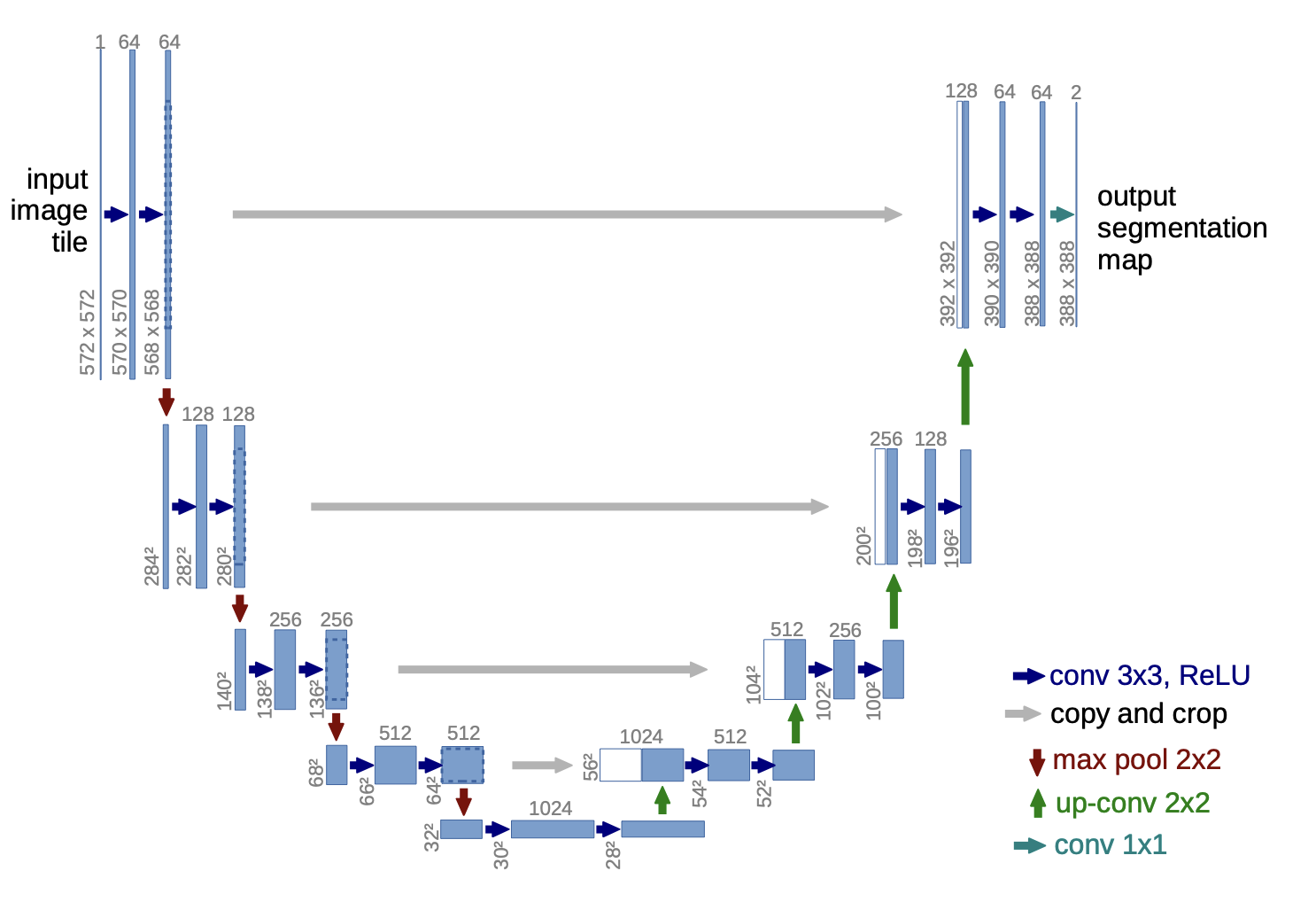
Above: Image of U-Net’s Architecture. Image courtesy of Ronneberger et al.
U-Net examines individual pixels of training images and uses the information provided to decide whether or not other pixels in unfamiliar images are part of a cell. Through its segmentation process, the algorithm uses input or training images and predicts the location of all objects collectively and individual objects. This technology’s ability to “zoom in” and “zoom out” makes it ideal for cell counting; it can gather information about both the complete image and individual objects—like cells—with relative ease.
U-Net’s architecture can be split into two main processes or goals: encoding and decoding. The encoding and decoding processes are represented by the left and right halves of the map, respectively. Through the encoding process, U-Net gathers relevant features from the images and then decodes by upsampling and concatenating (the left and right layers are connected) until it creates a segmentation mask that displays where the cells are. The upsampling process is an especially crucial stage; the algorithm maps the smaller feature map onto the size of the original image. Once an assay-specific model is created through this process, U-Net can batch-process and label cells from many images, producing rapid results.

Above: Image of segmented images of fluorescently labeled cells and performance data from different neural networks, including U-Net. Image courtesy of Fisherman et al.
U-Net’s ability to extract relevant pixel information and locate cells with high accuracy will continue to accelerate the process of counting in cell culture assays, immunostaining experiments, blood tests, and more. Applying models of artificial intelligence and neural networks to biological settings has the potential to revolutionize healthcare. For instance, complete blood cell counting is a standard procedure for diagnosing many serious conditions, including leukemia, anemia, and infections. Ensuring these results are produced quickly and with high accuracy is critical for patient care.
U-Net and similar technologies are far from error-free, but when used correctly, they provide clinicians and researchers with reliable, fast results. Although they serve their purpose in situations with small amounts of data or tight budgets, maybe it’s time we bench the hemocytometers.